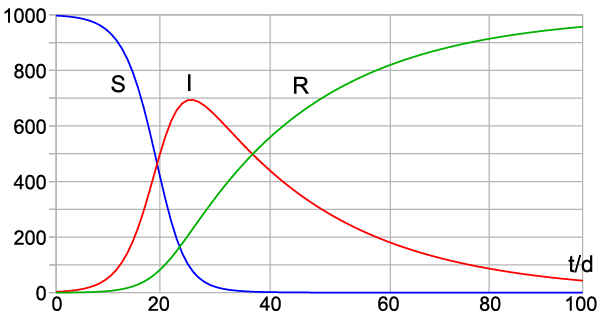
In my last post — from way too long ago, I really need to start posting more often — I defended the idea of epidemic modeling in general against a few know-nothing attacks. Along the way, I described the popular IHME model and how it was constructed. A few days later, an article by Sharon Begley at the online news magazine STAT reported on several criticisms of the IHME model:
A widely followed model for projecting Covid-19 deaths in the U.S. is producing results that have been bouncing up and down like an unpredictable fever, and now epidemiologists are criticizing it as flawed and misleading for both the public and policy makers. In particular, they warn against relying on it as the basis for government decision-making, including on “re-opening America.”
Unlike some of the nuttier tweets I quoted in my previous post, these criticisms come from the epidemiological community.
“It’s not a model that most of us in the infectious disease epidemiology field think is well suited” to projecting Covid-19 deaths, epidemiologist Marc Lipsitch of the Harvard T.H. Chan School of Public Health told reporters this week, referring to projections by the Institute for Health Metrics and Evaluation at the University of Washington.
I don’t want to fall into the fallacy of appeal to authority, but since most of us are not expert enough to understand the subject completely ourselves, authority certainly helps make the criticisms more credible. But there are other reasons to take the criticisms in the STAT article seriously.
Credible scientific criticism tends to have certain recognizable characteristics. Most importantly, in order to credibly criticize a piece of science, you have to actually understand the science. The great scientific revolutionaries, such as Albert Einstein, thoroughly understood the system they were seeking to overthrow. Failing to meet this obligation is a common problem with cranks and people driven by ulterior motives.
For example, when the Milken Institute School of Public Health came out with their 2018 study estimating that hurricane María caused 3000 deaths in Puerto Rico, many Trump opponents said it proved the Trump administration’s relief effort was shoddy and ineffective. Some of Trump’s supporters responded with ill-informed attacks on the study, several of which I described in my post on the subject at the time. But what neither side seemed to realize is that the study did not make any claims about the effectiveness of the Trump Administration’s relief effort. It wasn’t designed for that purpose and didn’t have access to the kind of data needed to assess the relief effort. The authors made this clear in their report. Obviously, most of the loud mouths on either side hadn’t actually read and understood how the study worked or what it was intended to discover.
That last part is important. In the absence of clear errors in the science, math, or data wrangling, most studies have a certain basic level of internal correctness. The real question is whether the methodology and data are suitable for the purpose for which the results are used.
Some years ago, the FBI reported statistics showing that most murder victims are killed by people they know. Advocates of stricter gun control leaped on this finding to argue that having a gun in your home would make you more likely to kill a family member than a criminal. But if you looked at how the FBI assembled the data, they included instances of criminals and gang members killing each other as crimes where the victims were killed by people they know. Because of this, the FBI report wasn’t very useful for answering questions about the advisability of having a gun in the home.
(I’m not sure, but I think the FBI was gathering this data because common murder investigation techniques often assumed a connection between victim and perpetrator, but police departments were seeing an increase in murders by strangers, such as serial killers, which required a different approach.)
This can be difficult to get right, and as with most things, the accuracy of understanding of scientific information tends to drop off as we get further and further from the source, and in the worst case can get pretty bad.
- The scientists who actually performed the study usually have a good grasp of its limitations.
- The big-shot scientist whose name is first on the paper might overstate the importance a bit.
- The university or corporate press release will probably get the basic idea right, but they’ll overstate the importance of the result and ignore the nuances and limitations.
- The press will focus on the most sensational aspects of the press release.
- Pundits and politicians will use the press accounts to support their prior beliefs and policies.
- Fringe bloggers and tweeters and political hacks on social media will take the resulting nonsense and pile more nonsense on top.
It doesn’t have to be like that, of course. At any step along they way, the people involved could go back and carefully read the original study paper, thus undoing much of the damage of this scientific game of telephone. (It’s what I try to do. Or failing that, I try to read accounts in the scientific press by reporters who have read the original material and can put it in a context I can understand.) Unfortunately, by the time the careful reading gets out, the crazy has been around the world.
The STAT article links to an article in the Annals of Internal Medicine which argues that the IHME model is not useful for planning:
The IHME projections are based not on transmission dynamics but on a statistical model with no epidemiologic basis. Specifically, the model used reported worldwide COVID-19 deaths and extrapolated similar patterns in mortality growth curves to forecast expected deaths. The technique uses mortality data, which are generally more reliable than testing-dependent confirmed case counts. Outputs suggest precise estimates (albeit with uncertainty bounds) for all regions until the epidemic ends. This appearance of certainty is seductive when the world is desperate to know what lies ahead. However, the underlying data and statistical model must be interpreted cautiously. Here, we raise concerns about the validity and usefulness of the projections for policymakers.
In just that single paragraph, the authors demonstrate that they understand how the IHME model works, and they argue that the model has little to offer for creating policy. The AIM article goes on to list six major areas of concern with the model. I’m not going to quote them — you can read the whole thing if you’re interested — but the article makes a lot of observations about the model in a relatively small space.
That brings me to another point: Credible criticism of science involves discussion of the actual science. For example, the issue of experimenter bias is obviously important, and studies by scientists with a history of bias or conflicts of interest should certainly be scrutinized carefully. However, it drives me nuts when pundits attack scientific studies by making ad hominem accusations of “bias” against the authors — because they’re paid by big oil, or because they donated money to the Clintons, or whatever — without backing it up with evidence from the study.
If you think a scientist is biased or has a conflict of interest, that’s definitely a fair thing to bring up when they’re expressing an opinion as an expert. But if you’re criticizing a scientific study (or experiment or model), you have to do better than that. If your argument is that the scientist’s bias has influenced the outcome of the study, then you should be able to point to the bias in the study. Proper scientific studies are reported out in the open, with detailed descriptions of their methodology, data, and analysis. If you think the scientists mucked it up, you should be able to point out where they made their mistakes.
This isn’t a hard thing to do if you’re familiar with the science. In a study performed by surveying people, you could criticize the choice of community from which subjects were drawn, or of how members are chosen from the community — using voting records, driver’s licenses, or commercial address lists will select for different kinds of people. You can argue that the people who agree to take part in the study are not representative — e.g. people willing to answer a stranger’s questions about their sex lives may be more likely to have unusual sex lives. You could argue that the survey questions have been misinterpreted by the subjects, or that the answers were misinterpreted by the scientists. You could argue that the wrong data sets were used, or that the data was mis-coded by the observers. If the study involves subjective judgement — e.g. doctor’s assessments of patient health — then you could argue that their judgment was influenced by their prior beliefs about the subject under study. The list of possibilities goes on and on, including lots of “gotcha” problems that are specific to a particular type of science. Many scientific studies talk about these issues openly in the interest of full disclosure.
I’m not saying you need have to have iron clad proof of a mistake or misrepresentation to establish bias, but if you are arguing that a scientific study is are biased, then you should be able to identify specific choices that you believe the scientists made because they were biased. That is, if you say the study is wrong (because of bias or any other reason), then you should be able to point out the part that is wrong. This is not a lot to ask.
(Of course, this isn’t always possible with every study. In the worst case, some papers are just poorly written, and you can’t critique how the study was done because the scientists didn’t describe it well enough, or it depends on data that is unavailable. This may prevent you from pointing to the part that is wrong, but it’s also a fair criticism to point out that the study is hard to evaluate, or that its conclusions are poorly supported.)
The authors of the AIM paper aren’t making accusations of bias, but they do specifically identify parts of the model they believe to be incorrect. They are specific about the science, the methodology, and the applicability of the model.
Others experts, including some colleagues of the model-makers, are even harsher. “That the IHME model keeps changing is evidence of its lack of reliability as a predictive tool,” said epidemiologist Ruth Etzioni of the Fred Hutchinson Cancer Center, home to several of the researchers who created the model, and who has served on a search committee for IHME. “That it is being used for policy decisions and its results interpreted wrongly is a travesty unfolding before our eyes.”
Movie director Jean-Luc Godard famously said, “In order to criticize a movie, you have to make another movie.” I don’t think I agree when it comes to movie criticism, but that is how science works. If you think a theory is wrong, pointing out weaknesses will only get you so far, because being wrong is not the same as being useless. As statistician George Box puts it, “All models are wrong, but some are useful.” So the best way to criticize a scientific theory is to offer a more useful theory. And the best way to criticize an epidemic model is to propose a more useful epidemic model.
The STAT article suggests two models that are arguably more useful. The first is a compartmental model from Dandekar and Barbastathis at MIT that takes into account the effects of quarantine measures. Compartmental models split the population into compartments representing different states of the disease for a person — e.g. Susceptible, Infected, and Recovered — and specify equations that describe how people move between the compartments.
The MIT model is grounded in epidemiological principles that have been understood for about a century, and which have been successfully used to predict things like annual influenza in the U.S. It is arguably a better model than the relatively simple IHME model, which just fits numbers to a curve. But is the MIT model accurate?
The model predicted U.S. cases would plateau at about 650,000 cases in mid April, which seemed pretty accurate at the time the STAT article was published. I’ve been lazy in writing this post, however, so I can see that the U.S. is now estimated to have about 860,000 active cases (over a million infected and about 150,000 recovered) as of the end of April, so the model seems to have missed. On the other hand, reporting of recoveries seems to be very delayed, so maybe not by much.
(The second model is a “data-driven” model by Huang, Qiao, and Tung, which sounds like a variation of the approach taken in the IHME model but, to be honest, the methodology goes over my head. I don’t really understand what they’re doing, so I can’t say much about it. This model also seems to have under-predicted the scope of the epidemic, with a mid-April peak and a final total of about 700,000 cases in this wave.)
Regardless of how these other models turned out, the STAT article didn’t just throw shade on the IHME model, it offered alternatives. That’s important because figuring out public health policy for fighting a pandemic is a plan for the future, and as I’ve said before, every time you plan for the future, you are basing your plan on a model. You have some mental idea of how the world works, and how you want to change it. So if you’re going to criticize the current model, you should probably be prepared to offer a better one.
This is why I’m weary of people who make angry claims that the science used to make Covid-19 policy decisions is wrong. Of course it’s wrong. All models are wrong. But that doesn’t mean it isn’t useful. And useful is a relative concept. Even if all you have is poor models, one of them is going to be the most useful model you’ve got.
You think the accepted Covid-19 case fatality rates are wrong? Fair enough, it’s certainly possible, and we can talk about it. But if you’re going to get angry at policy makers for using the accepted numbers, then you need to explain what numbers you think they should be using instead. You may indeed have found a flaw in the model, but if you can’t offer a better model, then you have no right to be angry at people for using the best model they have, at least until a better one comes along.
Addendum:
Here are some resources I found at FiveThirtyEight that might be useful:
[…] explain what happens next, I’d like to go back to something I skimmed over in my earlier post about a STAT magazine article that mentioned a Covid-19 model by Dandekar and Barbastathis at MIT. […]